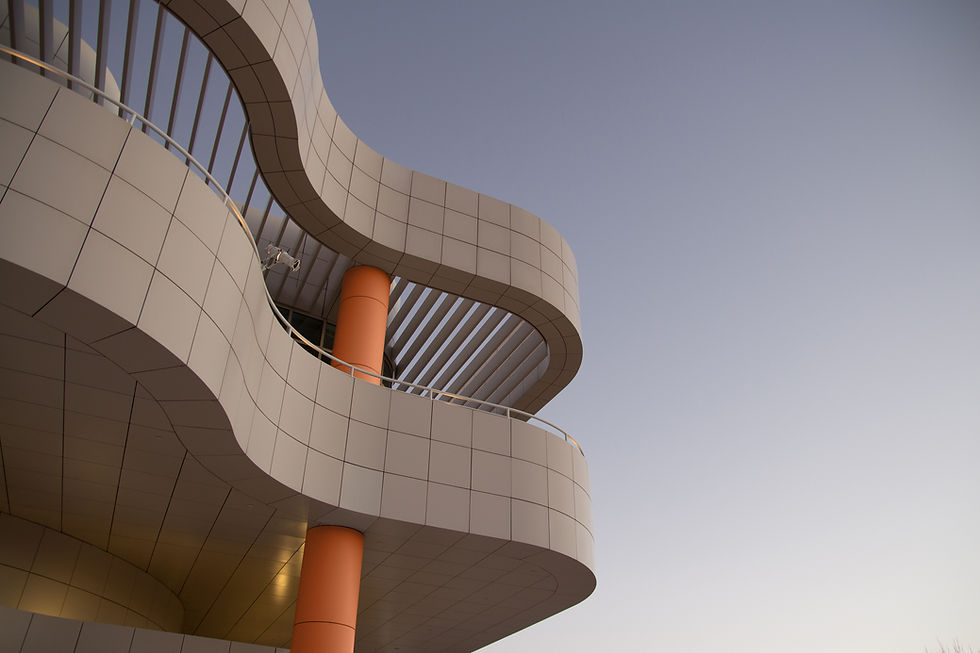
Advancements in AI-Based Machine Vision
Sep 25, 2024
4 min read
2
6
0
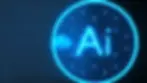
The integration of artificial intelligence (AI) into machine vision systems is transforming the landscape of manufacturing, particularly in quality control. Traditional vision systems relied on rule-based algorithms and basic image processing techniques, but recent advancements in deep learning and AI are pushing the limits of accuracy, adaptability, and efficiency. This post explores how AI-based machine vision systems are enhancing quality control and inspection processes in modern manufacturing environments.
1. The Evolution from Rule-Based to AI-Driven Vision Systems
Traditional machine vision systems were heavily dependent on predefined rules, fixed lighting conditions, and hard-coded algorithms to detect defects or measure components. Although effective in controlled environments, these systems often struggled with variability in product appearance, lighting conditions, and perspective shifts.
AI-driven systems, particularly those based on deep learning models like Convolutional Neural Networks (CNNs), have overcome many of these limitations. By training on large datasets, AI models can now recognize complex patterns, generalize across varying conditions, and make more accurate decisions, even when the visual environment changes. This adaptability is critical in high-speed, high-precision manufacturing lines where variability is inevitable.
2. CNN-Based Defect Detection and Classification
Convolutional Neural Networks (CNNs) have become the backbone of modern machine vision systems due to their superior ability to extract features from images. In a typical quality control process, CNNs can be trained to:
Detect Defects: CNNs can detect surface defects such as scratches, dents, or discoloration that may not be immediately visible to the human eye. These networks automatically learn to differentiate between acceptable variations in products and actual defects by analyzing thousands of labeled images.
Classify Anomalies: Beyond simple defect detection, CNNs can categorize defects into various types, providing detailed insights into production issues. For example, in automotive manufacturing, CNNs can classify different types of welding defects or material inconsistencies, helping to improve the production process and minimize waste.
Edge Detection and Contour Tracking: CNNs are highly effective at detecting edges and tracking contours in real-time, essential for quality assurance in industries like semiconductor manufacturing or electronics, where precise measurements are crucial.
3. AI for Anomaly Detection and Predictive Maintenance
While defect detection is critical, anomaly detection and predictive maintenance add another layer of intelligence to machine vision systems. AI-based anomaly detection leverages unsupervised or semi-supervised learning to identify outliers in a dataset. Unlike supervised learning, where the system is trained to recognize specific defects, anomaly detection algorithms learn the "normal" state of the product and flag deviations in real-time.
This approach is particularly valuable in scenarios where defects are rare or unpredictable, such as high-tech electronics manufacturing or pharmaceutical production. Additionally, combining AI-based machine vision with IoT sensors allows manufacturers to perform predictive maintenance on machinery, avoiding downtime and reducing repair costs by predicting when a machine or part is likely to fail.
4. Integration of AI in 3D Vision for Precision Inspection
3D vision systems, integrated with AI, are becoming increasingly prevalent in industries requiring high precision, such as aerospace and medical device manufacturing. 3D vision systems create depth maps of objects using techniques such as laser triangulation, time-of-flight, or structured light. When combined with deep learning algorithms, these systems can:
Analyze Surface Geometry: AI-based models can detect deformations, measure surface roughness, or ensure components meet exact geometrical specifications.
Part Alignment and Positioning: In robotic assembly lines, 3D AI vision helps robots correctly align and position parts, ensuring that complex assemblies are executed flawlessly.
5. AI-Enhanced Barcode Reading and OCR
Optical Character Recognition (OCR) and barcode reading are also seeing improvements with the adoption of AI. Machine vision systems using deep learning algorithms can now read damaged or low-contrast barcodes more reliably than traditional methods. In scenarios where parts are moving at high speeds or are partially obscured, AI-based vision systems significantly improve read rates and accuracy, enabling better tracking and traceability in manufacturing processes.
6. Challenges and Future Directions
Despite these advancements, AI-based machine vision systems face several challenges:
Data Requirements: Deep learning models require large amounts of labeled training data. In certain manufacturing scenarios, obtaining such data, especially for rare defect types, can be difficult.
Real-Time Processing: AI-based systems, particularly 3D vision systems, require significant computational power to perform real-time processing, which may lead to higher hardware costs.
Generalization: While AI models excel at specific tasks, generalizing across multiple product lines or adapting to significant environmental changes remains a challenge. Research is ongoing to develop more flexible AI models capable of handling a wider variety of conditions without extensive retraining.
Looking ahead, the integration of AI with other technologies such as edge computing, cloud processing, and 5G networks will further enhance the capability of machine vision systems. Edge-based AI will allow for lower latency and reduced data transmission costs, making real-time AI inspection even more feasible. Meanwhile, Generative Adversarial Networks (GANs) and self-supervised learning techniques are expected to further reduce the data requirements for training models, enabling more widespread adoption in smaller manufacturing environments.
Conclusion
AI-based machine vision systems are transforming quality control in manufacturing by providing unparalleled accuracy, adaptability, and intelligence. As these technologies continue to evolve, their impact on industrial automation will only grow, leading to higher efficiency, reduced waste, and better product quality across diverse industries.
Â